You’re about to launch a brilliant, innovative product and everyone is going to love it. Of course they are – they told you so! This is what many companies tend to think, but unfortunately the gap between expected and actual consumer behaviour is often big. In fact:
- Only 3% of new consumer packaged goods reach the $50 million sales benchmark of a high success launch during their first year.
- A whole 75% of new launches make less than $7.5 million in that time.
- And even if a product has a good start, many companies struggle to maintain initial success.
A few out of numerous possible explanations for a failed launch are introducing the product too early when it is not completely flawless, the company not being prepared for fast growth, not giving consumers enough information about new product categories, or launching something when there is no market for it – when there’s a gap in the market, it might be because there’s no market in that gap.
What do you need to know?
Successful sales of a product do not only depend on a good launch. There are many critical and persistent factors to be aware of with new (as well as well-established) products and services. Examples of those are customers’ willingness to pay, price sensitivity, competitors’ offers, branding, packaging, or advertising. But how can you take into account so many variables and predict people’s preferences? Are people’s choices even predictable?
One method commonly used to explore customer attitudes is to send out a survey, directly asking possible buyers’ opinions and preferences. This will give your company a good idea in which direction to move. Since people’s intentions often don’t end up aligning with their actions (Ajzen, 1991), simply surveying their declared attitudes will likely not give you the full picture. – ‘Tell us how much you’d be willing to pay’ never matches reality. (However annoying that may be.)
Imagine you are about to launch a healthy superfood granola bar. Respondents of your survey love the idea, show clear interest in healthy food choices and intend to buy your product. Next weekend at the discount grocery store they still end up buying the granola bar they always buy – because when declaring their intentions, they weren’t aware of actual behavioural control. Habits (we tend to buy the same items), external influences (your granola bar is only available at specific stores), and situational states (spontaneous hunger and craving for comfort food) widen the gap between attitudes and behaviour.
So if choices depend on so many different and unforeseeable factors and survey respondents cannot even predict their own choices, how do we find out if people will love your product, what they will love about it and when they will love it most? The method you are looking for is ‘conjoint analysis’.
Conjoint analysis is a statistical method and its opportunities go far beyond the scope of a classic survey. With conjoint analysis it is possible to determine how people value the different features and attributes of which a product or service consists. Its ability to evaluate many separate aspects of a product and turn that into one robust, predictive ranking is beyond important for new product/service launches. (Imagine a smartphone and the features and combinations – battery life, brand, screen size, operating system, price, and more, ranked by combinations from most desirable to least.)
Participants are confronted with choice scenarios and simply have to make a number of successive decisions (illustration below presents one choice set). The products or services between which they are deciding are described by a selection of attributes. All attributes are presented with different levels and the combination of levels changes according to a mathematical algorithm. After a participant made enough choices the programme calculates the relative importance of each attribute depending on how dominant it was in participants’ decision making.
Figure 1. Illustration of choice set as viewed by participants
Different versions of conjoint analysis focus on different applications. Apart from the generic conjoint, other types include for example a brand-specific version, a predictive product test, a Gabor-Granger version, and a Van Westendorp version. All types together offer testing of customers’ willingness to pay, price sensitivity, competitors’ offers, branding, packaging, or advertising.
With this full repertoire, conjoint analysis offers the advantage to be tailored to a company’s individual needs and issues. The opportunity to use physical objects in testing, to choose individually relevant attributes and levels, to estimate real life trade-offs and to uncover consumers’ implicit preferences makes it unique and valuable for anyone thinking about predicting the future.
There really is a crystal ball.
References
Ajzen, I. (1991). The theory of planned behavior. Organizational behavior and human decision processes, 50(2), 179-211.
Conjoint.ly. (2018). Conjoint Analysis. Retrieved from https://conjoint.online/conjoint-analysis/
Hall, J. S. (2014, August 01). Why Most Product Launches Fail. Retrieved from
https://hbr.org/2011/04/why-most-product-launches-fail
Shah Alam, S., & Mohamed Sayuti, N. (2011). Applying the Theory of Planned Behavior (TPB) in halal food purchasing. International Journal of Commerce and Management, 21(1), 8-20.
The Power of Conjoint Analysis. (2017, August 16). Retrieved from
https://www.questionpro.com/blog/power-conjoint-analysis/
Image c/o https://flic.kr/p/VESPx6
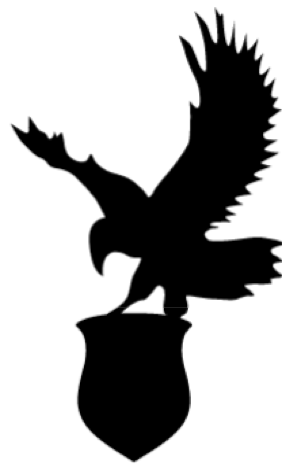
For more on this speak with us, or have a look at our capabilities
Also, as co-founders and supporters of the London Behavioural Economics Network, join the Meetup group and Facebook group for more details and events
Related Posts
August 13, 2023
Money (but not called that, and how it changes behaviour).
Advertising agencies make a lot of…
August 1, 2023
Reading ease is not as easy as all that – comprehension shows the way
It's easy to make text readable, right?…
July 20, 2023
The ‘Hollywood hello’ – and the importance of context when communicating
When communicating we all like to be as…